by Kit Walsh
Electronic Frontier Foundation
Reprinted under Creative Commons License
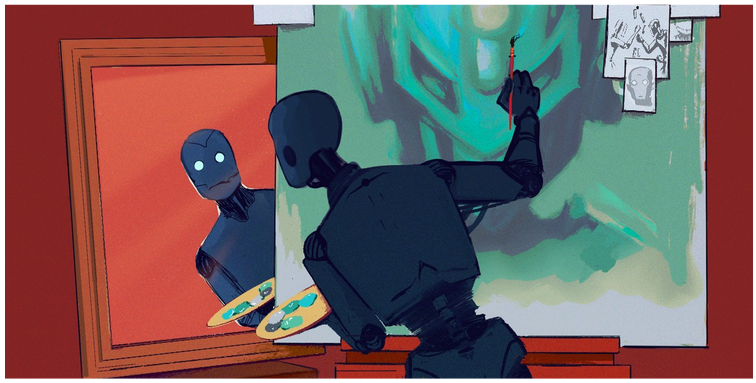
Artists are understandably concerned about the possibility that automatic image generators like Stable Diffusion will undercut the market for their work. We live in a society that does not support people who are automated out of a job, and being a visual artist is an already precarious career.
In this context, it's natural to look to copyright law, because copyright is supposed to help ensure that artists get paid for their work. Unfortunately, one copyright theory advanced in a class-action lawsuit by some artists against Stable Diffusion is extremely dangerous for human creators. Other theories—both in that lawsuit and another suit by Getty Images—propose to alter and expand copyright restrictions in ways that would interfere with research, search engines, and the ability to make new technology interoperate with old.
This legal analysis is a companion piece to our post describing AI image-generating technology and how we see its potential risks and benefits. We suggest that you read that post first for context, then come back to this one for our view on how the copyright questions play out under U.S. law.
Copyright law is supposed to embody a balance between giving artists a sufficient incentive to create, by granting them control of some of the ways their art can be used, and giving the public the right to build on and/or use that art in new and interesting ways. Here, the question is whether those who own the copyright in the images used to train the AI generator model have a right to prohibit this kind of use.
To answer that question, let's start with a few basic principles.
First, copyright law doesn't prevent you from making factual observations about a work or copying the facts embodied in a work (this is called the "idea/expression distinction"). Rather, copyright forbids you from copying the work's creative expression in a way that could substitute for the original, and from making "derivative works" when those works copy too much creative expression from the original.
Second, even if a person makes a copy or a derivative work, the use is not infringing if it is a "fair use." Whether a use is fair depends on a number of factors, including the purpose of the use, the nature of the original work, how much is used, and potential harm to the market for the original work.
Copyright and Training Sets
Here's how fair use would apply to AI art generation:
Step 1: Scraping the Images from the Web
Like copying to create search engines or other analytical uses, downloading images to analyze and index them in service of creating new, noninfringing images is very likely to be fair use. When an act potentially implicates copyright but is a necessary step in enabling noninfringing uses, it frequently qualifies as a fair use itself. After all, the right to make a noninfringing use of a work is only meaningful if you are also permitted to perform the steps that lead up to that use. Thus, as both an intermediate use and an analytical use, scraping is not likely to violate copyright law.
Step 2: Storing Information About the Images
In this step, the system analyzes the images and stores information about how the pixel arrangements correlate with words in the text annotations.
The Stable Diffusion model makes four gigabytes of observations regarding more than five billion images. That means that its model contains less than one byte of information per image analyzed (a byte is just eight bits—a zero or a one).
The complaint against Stable Diffusion characterizes this as "compressing" (and thus storing) the training images, but that's just wrong. With few exceptions, there is no way to recreate the images used in the model based on the facts about them that are stored. Even the tiniest image file contains many thousands of bytes; most will include millions. Mathematically speaking, Stable Diffusion cannot be storing copies of all of its training images (for now, let's put a pin in the question of whether it stores a copy of any of them).
So the model isn't storing copies. But is it generating and storing infringing derivative works of all of the images in the training data?
Probably not, for at least three reasons:
First, a derivative work still has to be "substantially similar" to the original in order to be infringing. If the original is transformed or abridged or adapted to such an extent that this is no longer true, then it's not a derivative work. A 10-line summary of a 15,000-line epic isn't a derivative work, and neither are most summaries of books that people make in order to describe those copyrighted works to others.
Second, copyright doesn't grant a monopoly on a genre or subject's tropes and motifs, including expressive elements like wavy lines to denote shaking, giving animals more human facial expressions, and similar common—even if creative—choices. What's more, copyright does not apply at all to non-creative choices—like representing a cat as having four legs and a tail. Much of the information stored by and produced by an AI art generator falls into these categories.
Third, the amount of copyrightable expression taken from each original image in the training set could be considered "de minimis," a legal term that means "too minimal to qualify as infringing."
Even if a court concludes that a model is a derivative work under copyright law, creating the model is likely a lawful fair use. Fair use protects reverse engineering, indexing for search engines, and other forms of analysis that create new knowledge about works or bodies of works. Here, the fact that the model is used to create new works weighs in favor of fair use as does the fact that the model consists of original analysis of the training images in comparison with one another.
The class-action lawsuit against Stable Diffusion doesn't focus on the "outputs," (the actual images that the model produces in response to text input). Instead, the artists allege that the system itself is a derivative work. But, as discussed, it's no more illegal for the model to learn a style from existing work than for human artists to do the same in a class, or individually, to make some of the same creative choices as artists they admire.
Moreover, AI systems learn to imitate a style not just from a single artist's work, but from other human creations that are tagged as being "in the style of" another artist. Much of the information contributing to the AI's imitation of style originates with images by other artists who are enjoying the freedom copyright law affords them to imitate a style without being considered a derivative work.
Step 3: Creating Output Images
Unlike the Stable Diffusion case, Getty Images's suit focuses on outputs, claiming that outputs are sometimes "substantially similar" to training data. Getty doesn't provide an example of this, apart from the presence of its watermark in some Stable Diffusion outputs.
It's not surprising that the complaints don't include examples of substantially similar images. Research regarding privacy concerns suggests it is unlikely it is that a diffusion-based model will produce outputs that closely resemble one of the inputs.
According to this research, there is a small chance that a diffusion model will store information that makes it possible to recreate something close to an image in its training data, provided that the image in question is duplicated many times during training. But the chances of an image in the training data set being duplicated in output, even from a prompt specifically designed to do just that, is literally less than one in a million.
This means that there exists, at most, a handful of rightsholders out there that might have a copyright claim. Thus far, neither lawsuit suggests the plaintiffs are in that category.
Of course, the statistical standards used in this research aren't the same as the legal standards used in copyright law, but we can nonetheless take them as informative, and they are consistent with the tiny amount of data per image the diffusion model stores.
To sum up: a diffusion model can, in rare circumstances, generate images that resemble elements of the training data. De-duplication can substantially reduce the risk of this occurring. But the strongest copyright suit against a diffusion-based AI art generator would likely be one brought by the holder of the copyright in an image that subsequently was actually reproduced this way.
As with most creative tools, it is possible that a user could be the one who causes the system to output a new infringing work by giving it a series of prompts that steer it towards reproducing another work. In this instance, the user, not the tool's maker or provider, would be liable for infringement.
What Would it Mean for Art if the Court Finds that Stable Diffusion Infringes Copyright?
The theory of the class-action suit is extremely dangerous for artists. If the plaintiffs convince the court that you've created a derivative work if you incorporate any aspect of someone else's art in your own work, even if the end result isn't substantially similar, then something as common as copying the way your favorite artist draws eyes could put you in legal jeopardy.
Currently, copyright law protects artists who are influenced by colleagues and mentors and the media they admire by permitting them to mimic elements of others' work as long as their art isn't "substantially similar" and/or is a fair use. Thus, the same legal doctrines that give artists the breathing room to find inspiration in others' works also protect diffusion models. Rewriting those doctrines could cause harm far beyond any damage Stable Diffusion is causing.
In our companion blog post, we explore some of the other consequences. In particular, we discuss who would likely benefit from such a regime (spoiler: it's not individual creators). We also discuss some alternative approaches that might actually help creators.
Done right, copyright law is supposed to encourage new creativity. Stretching it to outlaw tools like AI image generators—or to effectively put them in the exclusive hands of powerful economic actors who already use that economic muscle to squeeze creators—would have the opposite effect.
|